I often describe our society as “increasingly nonpermissive,” but what does this really mean? For former CIA officer Tony Mendez, the Soviet capitol city of Moscow was the quintessential nonpermissive environment — one in which CIA officers, or other embassy personnel, could assume with a high degree of certainty that they were under surveillance. Given the unrelenting scrutiny of the KGB, activities such as meeting with agents or picking up dead drops were essentially out of the question. The CIA and the officers at Moscow Station were able to develop new tradecraft, including sophisticated disguises developed with the aid of Hollywood makeup artists, to allow them to evade their KGB opponents and begin operating again. Mendez details this, and many other relevant tidbits, in his book The Moscow Rules.
However, as we move into the 21st century, nonpermissive environments have taken on a new meaning. The average person on the street in the United States or Western Europe might not need to fear being tailed by agents of the state every time her or she leaves home (yet), but the digital Panopticon is upon us for data monetization purposes, as well as for reasons of “public safety.” Pervasive CCTV camera deployments, social networking, and advances in the fields of high-performance computing, “artificial intelligence,” and machine learning are pushing us toward a dangerous future very similar to what Philip K. Dick described in The Minority Report.
In fact, for places like China, where facial recognition is already widely deployed, be it at restaurant kiosks for payment and ordering suggestions, or via surveillance cameras on the street corners, the world of The Minority Report is already here.
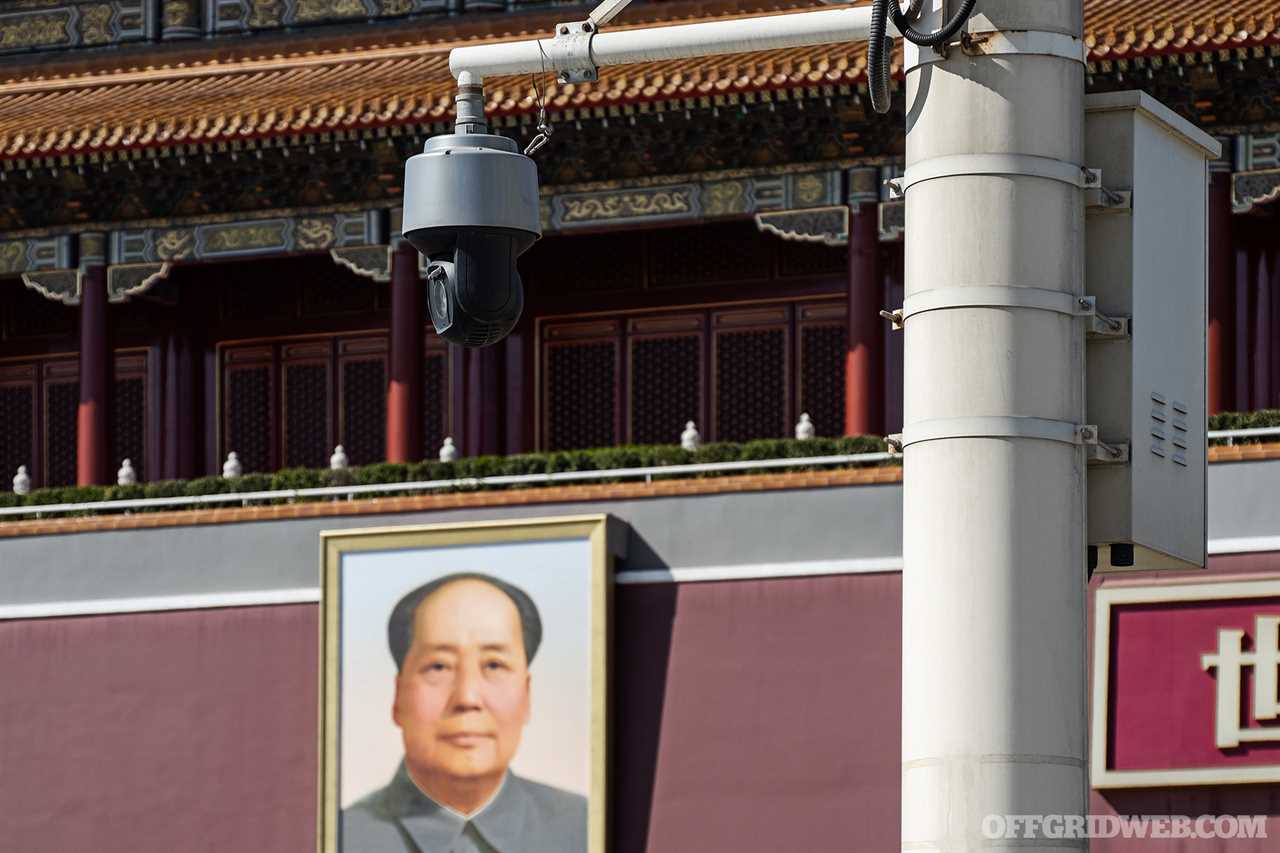
Above: Unsurprisingly, China has been a pioneer in the field of facial recognition camera systems. They provide the CCP with a fast and effective means of tracking down citizens who step out of line.
So, how does the technology work? Where might we encounter it today or in the future? And how, if at all, can we defeat it and maintain our privacy? Let’s discuss.
What Are Biometrics?
Biometrics refers to a set of technologies, generally used as security controls, which are predicated on that fact that individuals have certain universally unique characteristics. The first thing that might come to mind is fingerprints. Chances are that your smartphone or laptop has a fingerprint scanner and will allow you to log into the system with a simple scan rather than entering a password. As far as a measure of uniqueness goes, fingerprints are top-notch. There has never been a documented case of two people having the same fingerprints — not even identical twins. Theirs may be very similar, but they are, in fact, different.
Some other biometrics that can be used to uniquely identify an individual include:
- Iris of the eye
- Palm prints
- Voice prints
- The face
While facial recognition is what the majority of this article is about, it’s important to understand biometrics in general as they have a lot of things in common.
As a cybersecurity engineer by trade, I have a love/hate relationship with biometrics. In fact, what I view as their key disadvantage as an authentication factor is actually what makes them so dangerous from a privacy standpoint. That is, you can’t replace them once they’re lost. If I forget a password, or lose a CAC card, I have recourse. I can have my password reset or have the keys on the card revoked and a new one issued. Not so with my biometrics — they’re permanent.
And, unlike “something I know” (i.e., a password), or “something I have” (the CAC card), the “something I am” is always visible, and oftentimes left behind. So while biometrics are an excellent way to establish identity, I find the way they are actually leveraged as a replacement for passwords is very problematic.
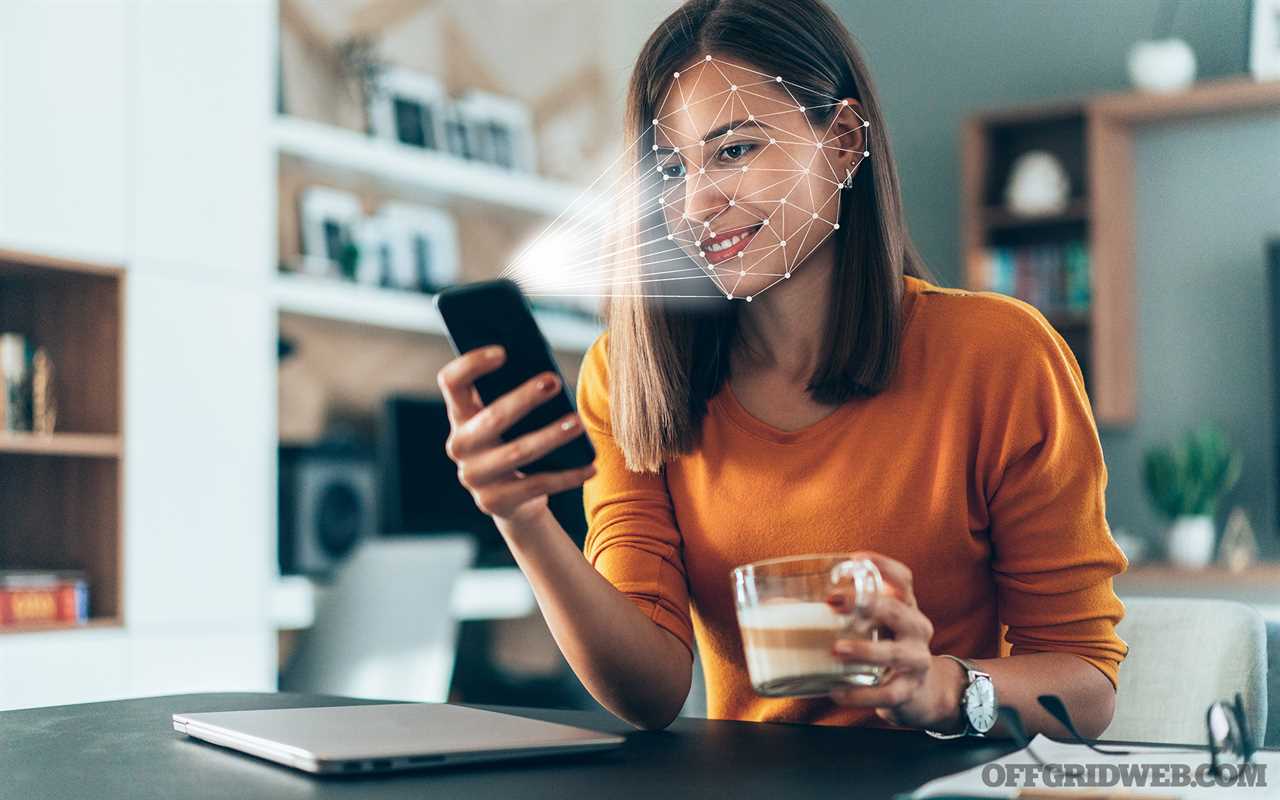
Above: Many of us use biometrics as a convenient way to unlock our devices. However, few consider the security implications of using an unchangeable feature — such as fingerprints or the face — instead of an easily changed password.
Biometric systems are also subject to two types of errors. Type I errors are false negatives, and Type II errors are false positives. In biometrics terms, these are called false rejection and false acceptance. The accuracy of a system is measured by the point where the false acceptance rate equals the false rejection rate. This is called the Crossover Error Rate (CER), and the lower the CER the better. Looked at another way, the lower the CER, the harder it is for the system to be tricked.
Understanding CER is key to understanding both whether it’s worth it to deploy a biometric security control, or how you might go about defeating one.
At a high level, all biometric systems have the same basic components:
- Some sort of sensor to take the data input (fingerprint or iris scanner, camera, etc.).
- A database containing all the enrolled data (pre-existing samples of the biometric, associated with an individual).
- A processing system that creates the mathematical model of the biometric during enrollment and is also capable of real-time processing inputs to match against the database.
If you have a modern smartphone, you’re likely familiar with the process of enrollment. If you have ever worked in a secure environment in government or even the private sector, you’re also likely to have gone through the enrollment process.
The sophistication of the input sensors is of utmost importance. In 2013, for instance, the Chaos Computer Club, a hacker group in Germany, demonstrated an attack against Apple’s TouchID which enabled them to gain access to someone else’s iPhone. By transferring the target’s fingerprint onto a gummy bear candy, they were able to give to trick the sensor into unlocking the phone. In 2017, another German security outfit, SYSS, demonstrated that they could bypass Windows 10’s facial recognition with a specially printed head shot of the spoofed user.
The next most important feature of the system is the enrolled dataset. When it comes to users of phones, computers, or even those granted access to a specific section of a building, that enrolled dataset is pretty small and focused. When scaling up for mass surveillance purposes, that dataset (and the processing power required) starts to grow exponentially.
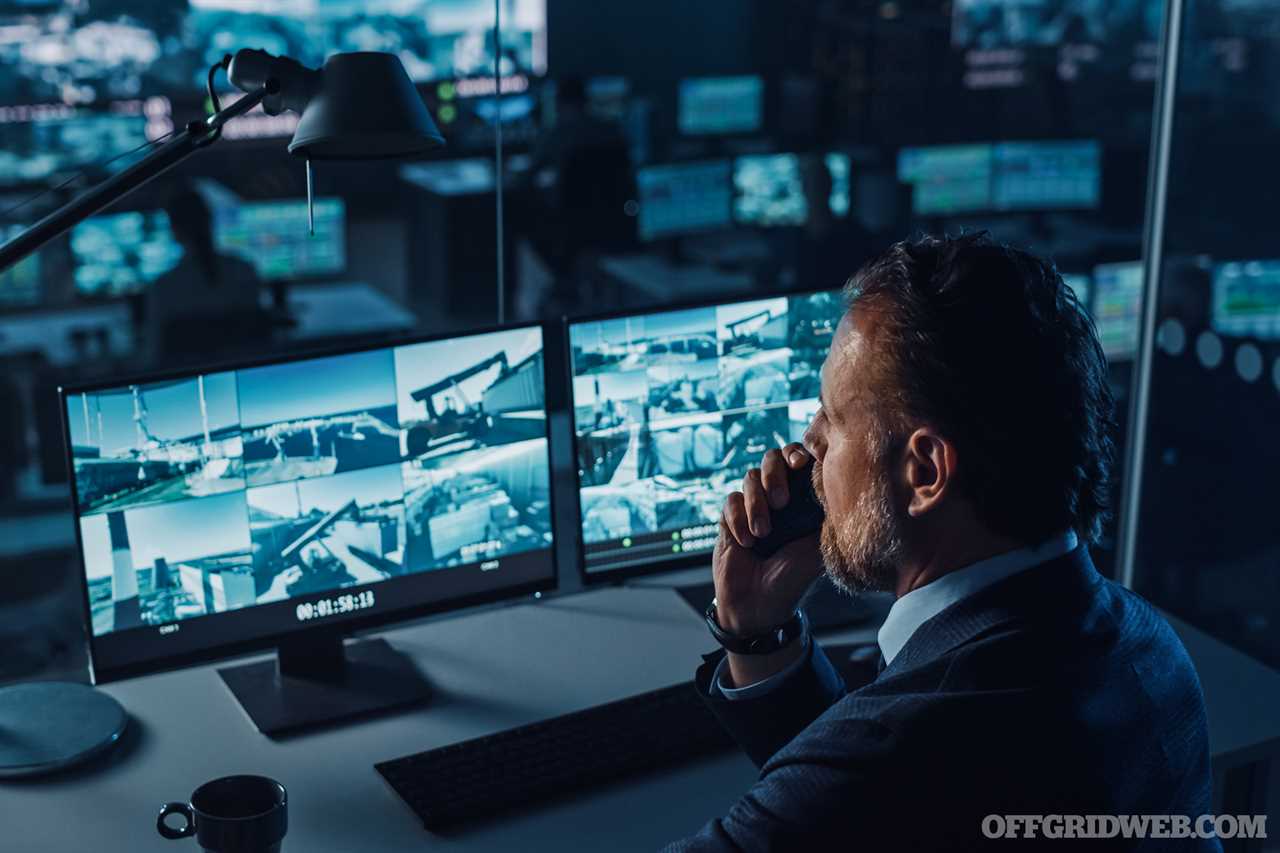
Above: Unlike humans monitoring security cameras, facial recognition systems never fall victim to distractions or fatigue. They leverage computing power to spot targets with superhuman speed and accuracy.
Facial Recognition: How it Works
Now that you know some basics about biometrics in general, let’s focus on facial recognition in particular. Facial recognition is composed of two major phases. The first is facial identification and the second is facial recognition. Identification is determining “do I see a face” and recognition is determining “do I know who this face belongs to.”
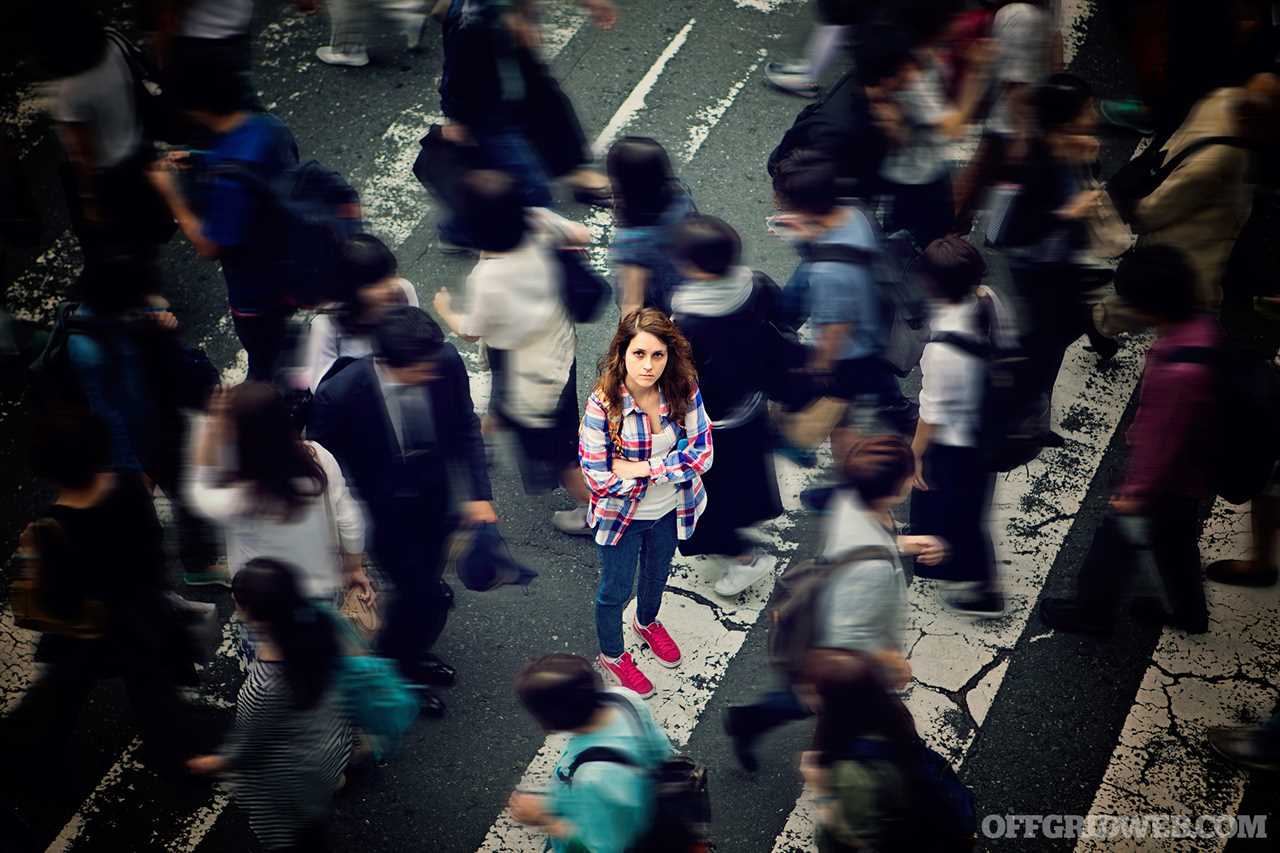
Facial identification requires cameras and a good mathematical model of what a face is or isn’t. Traditionally these cameras were visible light cameras, but the need to function in low-light, especially in surveillance purposes, as well as the need to get high-grade contrast, means many operate in the near-IR spectrum, like night vision devices do.
Facial recognition requires the system to have an enrolled dataset. How exhaustive the dataset needs to be is dependent on the application.
Understanding the two-phase process and their components is key to developing countermeasures, which we will discuss later. But why would people want countermeasures in the first place?
The wide-spread deployment of facial recognition technologies, driven by machine learning (ML) and “artificial intelligence” (AI) systems is, in terms of threats to a free society, second only to the adoption of a Central Bank Digital Currency (CBDC). And, just like CBDC, the masses have been conditioned over time to accept aspects of it, or its forerunner, in their lives under the guise of “cool” or “convenient.” Some examples include:
- Facial identification helping auto-focus the camera on your smartphone when you’re taking photos.
- Facial recognition helping to automatically tag “friends” when you upload your photos to social media.
- Facial recognition being used to unlock phones and computers.
Making it cool, fun, and convenient creates a situation where people actively, willingly, participate in feeding the data model. For years, people have been uploading photographs of themselves, friends, and family, to social media sites. These sites then introduced facial identification and allowed you to tag the face with who it belongs to. Eventually social media started offering to tag photos for you, which is fun and convenient, right? Well, it can do that because machine learning models were built and trained by people tagging photos.
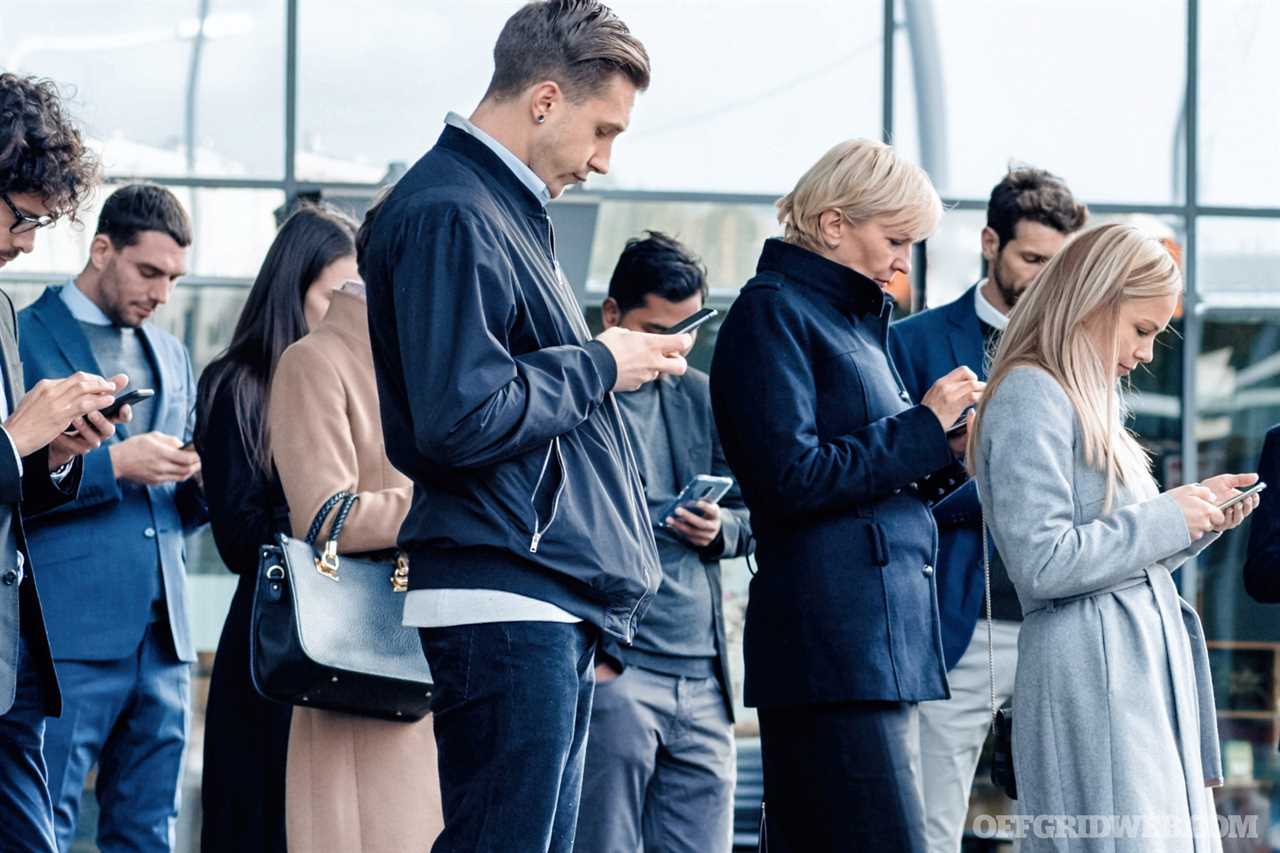
Above: Millions of photos are uploaded to social media and tagged every day. Facial recognition models are being perfected through this massive influx of data.
Think of all those photos of people, at different angles, in different lighting conditions, at different ages. If you wanted to build the perfect dataset for an automated facial recognition system, you couldn’t ask for a better one — certainly not the state DMV database or State Department’s database of passport photos.
But what about the model? Just having photos of individuals isn’t enough. Each image needs to be analyzed in order to build a mathematical model of that person’s face. These days, system designers are increasingly relying on technologies like convolutional AI to automate the creation of these models via processes which are opaque to them. However, in general facial recognition models are going to be based on the geometric relations between facial landmarks, such as:
- Distance between eyes, ears, etc.
- Breadth and length of the nose.
- Bone structure of the face (cheek bones, brow ridge, etc.)
Additionally, some of these measurements will be based on measured or inferred depth. These measurements require good lighting and contrasts in order to assess, a situation which has led to no small amount of controversy in recent years. In recent years, there have been no small number of cases where facial recognition technologies, deployed for various purposes, have been accused of being “racist,” either because the sensors have trouble with darker-skinned subjects, or because the training dataset for the machine learning algorithms is predominantly white or Asian.
Because this causes issues with applications that people want to work, such as device security or social media applications, these complaints tend to drive the state of the art in pushing facial modeling, advancing it in the general case.
The Facial Recognition Threat Right Now
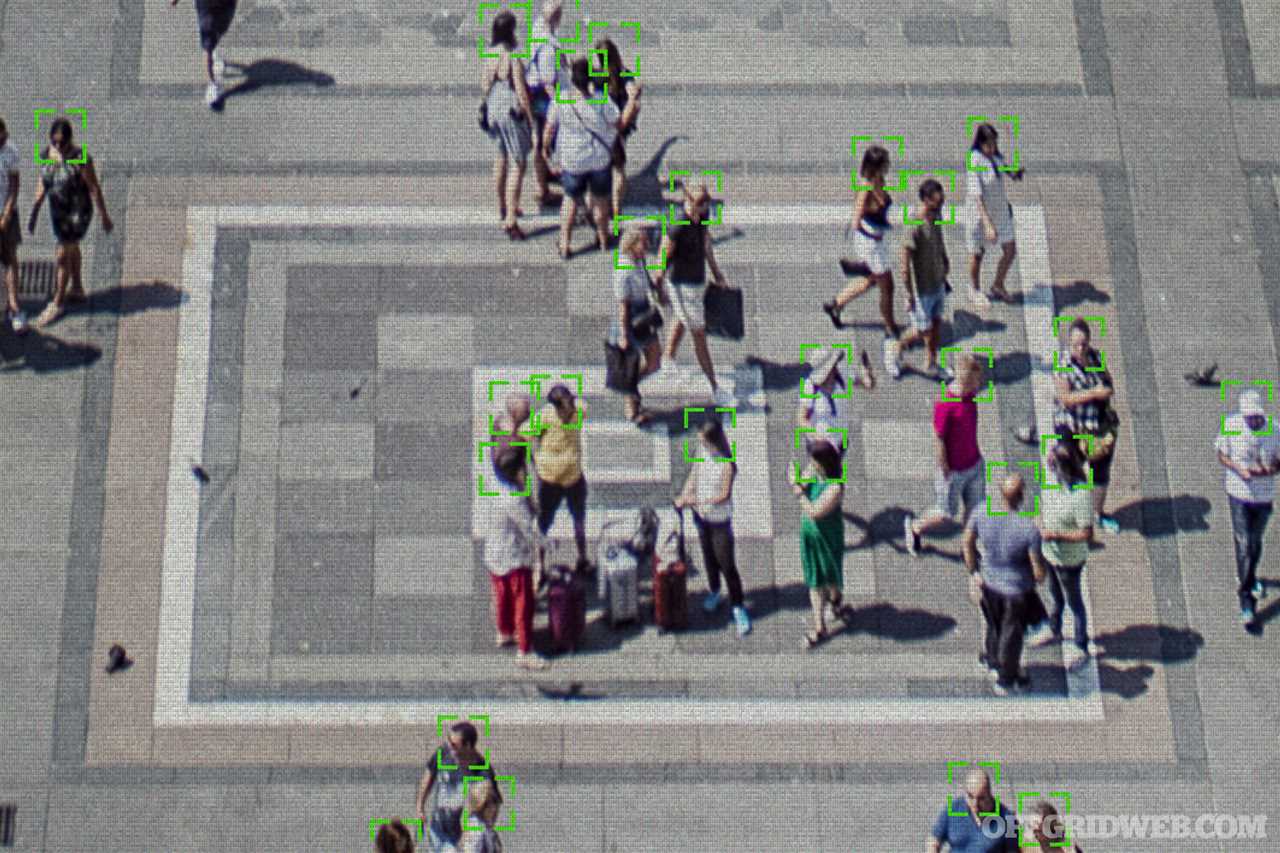
Above: Whether you’ve noticed it or not, facial recognition technology is already being used extensively in crowded public places, especially within countries that favor authoritarian control over citizens’ right to privacy.
In China, the future is now. Mass deployment of surveillance cameras hooked up to high-performance computing clouds, with massive datasets, provide an all-seeing eye. People caught merely jaywalking are identified and then put on digital billboards to humiliate them and force social conformity, all in keeping with their social credit system. Facial recognition is tied to digital ID and payment systems. You can go into a fast-food restaurant, walk up to the kiosk, be served, and have your account debited, all from facial recognition. Fun, cool, and convenient, right?
The much darker side is that while social justice warriors in the U.S. and Europe are misguidedly pushing to help make facial recognition technology better at identifying minorities, in China they have developed data models and algorithms which can identify, with a great deal of accuracy, the ethnicity of a person. This technology is being used specifically to target the frequently persecuted Uighur minority population in Western China’s Xinjiang province.
In the U.S., we have protections that China doesn’t have. When the first publicly documented case of police using facial recognition technology en masse came to light in 2001 at the Tampa-hosted Super Bowl, there was a wide-spread outcry about how it was a 4th Amendment violation. Of course, this was pre-Sept. 11, pre-PATRIOT Act, and before Snowden’s revelations that would make this seem like a blip. In the U.S. now, some cities have created ordinances banning the use of facial recognition technology, sometimes due to privacy implications, other times at least in part because of the seemingly disproportionately high false positive rate for minorities leading to incorrect identification and false arrests. (Boston, Massachusetts, and Portland, Oregon, for instance, rolled out their ordinances against facial recognition in 2020 so that police could not use it during the ongoing riots and protests).
However, there are areas of the U.S. where the rules don’t always apply. Borders and checkpoints are one. Automated immigration checkpoints comparing on-site snapshots to your passport photo are becoming well established in the U.S. and other rich countries, offering convenience for greater acceptance of the tech. There can be no doubt that facial recognition technology is being deployed in the surveillance systems of major airports as well.
The same mobile technology that was pioneered and rebuked two decades ago will continue to make appearances at major events and especially at protests. And even when real-time facial recognition isn’t in play, surveillance photographs can be compared to government and open-source data sets (all those photos you put on the internet) for identification. This tactic was heavily leveraged both by government employees and private-sector open-source intelligence (OSINT) analysts and digital sleuths after the events on January 6, 2021, for instance.
The Threat in the Future
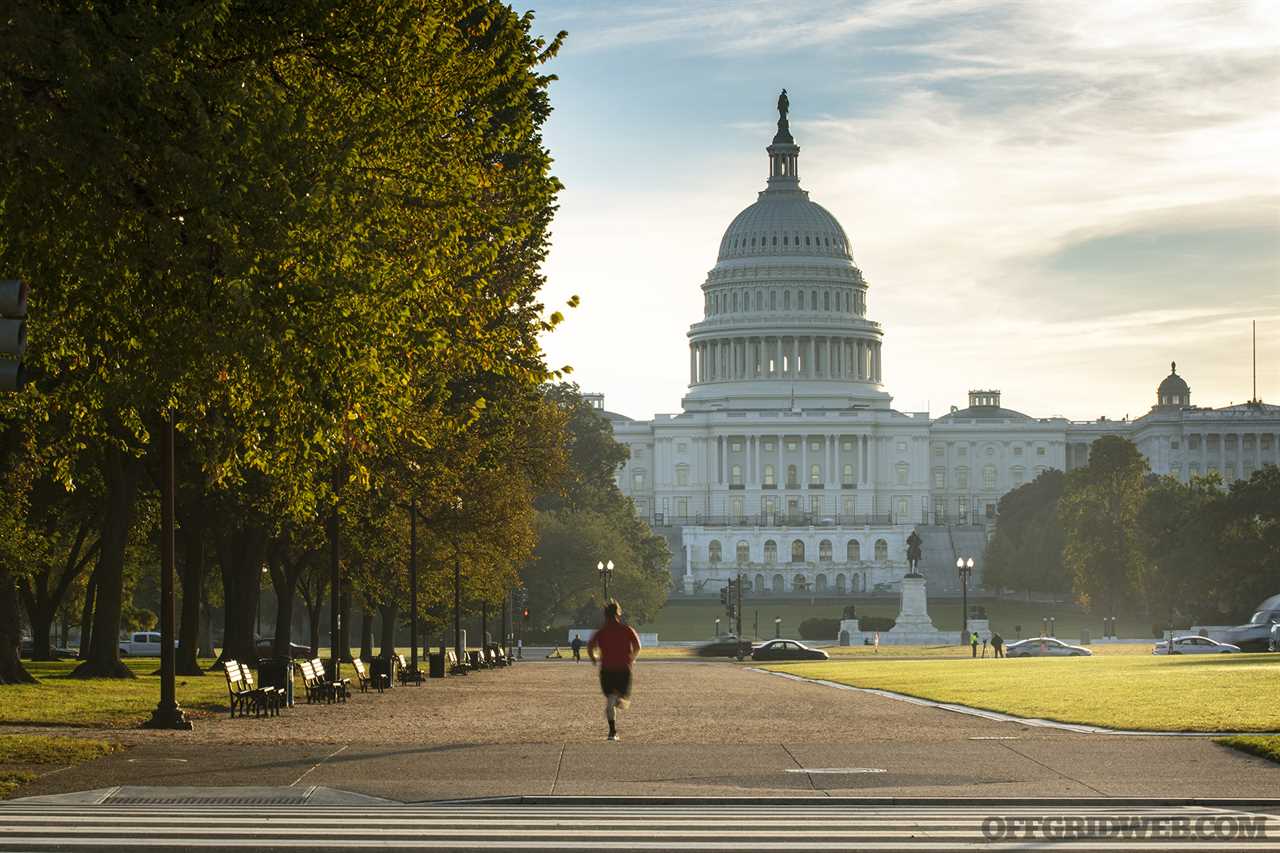
In the U.S., we’re highly suspicious of three-letter agencies hoarding and manipulating our sensitive data, but many of us hand that same data to social media and tech corporations without blinking an eye. Here, the threat of invasive facial recognition is less likely to come directly from the government and more likely to be privatized. As China is doing now, and as The Minority Report showed, we’re likely headed to a future where the profit-fueled surveillance we have long known in the online world will move to the real world. You’ll walk into a store, be identified, and then based on your likes and internet history, will be offered products in real time. Or, based on your Personal ESG score — a measurement of how environmentally friendly and socially conscious your lifestyle is perceived to be — you might even be told you can’t spend money there.
As the social acceptance of the technology grows until it becomes basic background noise like flushing toilets and flipping light switches, there’ll be fewer and fewer legal challenges, and eventually government surveillance will step up as well. Via “public-private partnerships” in the name of “public safety,” we’ll find the lines increasingly blurred.
At least, that’s my projection.
What About Countermeasures?
Some systems are going to be harder to trick than others. How hard is going to be a function of how good the hardware is, how exhaustive the database is, and how sophisticated the model is. Saying for sure what will or won’t work is therefore hard. However, with some experimentation and research, I have a few things that I know will defeat some systems and may have success against others.
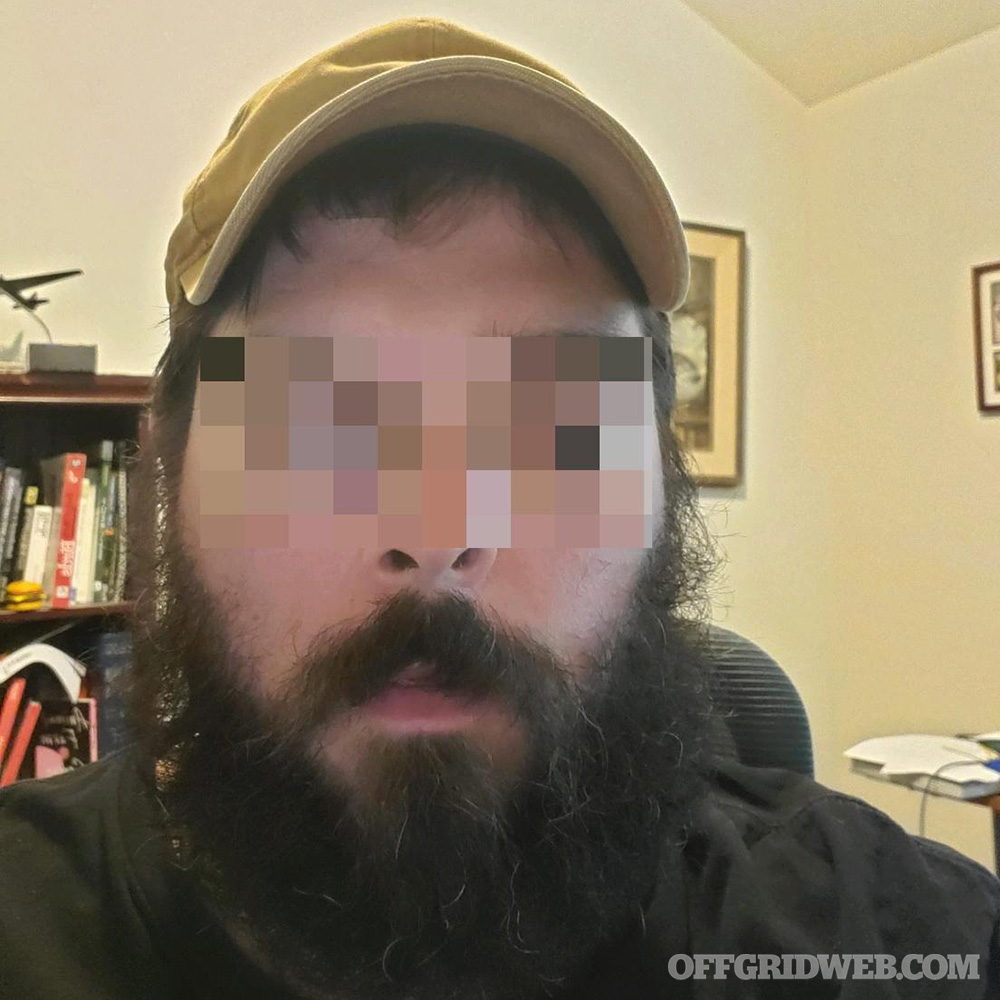
Above: The Android application ObscuraCam can quickly edit out faces and other distinguishing marks. This is perfect for countering facial recognition and methods OSINT analysts might use.
Online Countermeasures
With regards to online countermeasures, the goal is to deny the creation of a good data model of your face. This can basically be broken down into two tactics:
- First, is adversarial modeling. In machine learning, this essentially means spoiling the dataset with lies. You operate an account as yourself, or otherwise upload photos, but the photos are not of you. You then tag those photos as you, so the data model doesn’t associate your face with your person.
- The second tactic, and one that’ll bring you much joy in your life, is to simply avoid playing the game. Get off social media. Spoil all your data, then delete your account. If you never had social media, all the better. Ask your family and friends not to upload photos of you. If they must, blur out the photos.
If you must swap photos online, use secure or covert communications applications to do it, and spoil the photos directly. Applications like ObscuraCam can take advantage of facial identification and pixelate or otherwise redact the photo when you take it. You can also use it to quickly obscure any other identifying information.
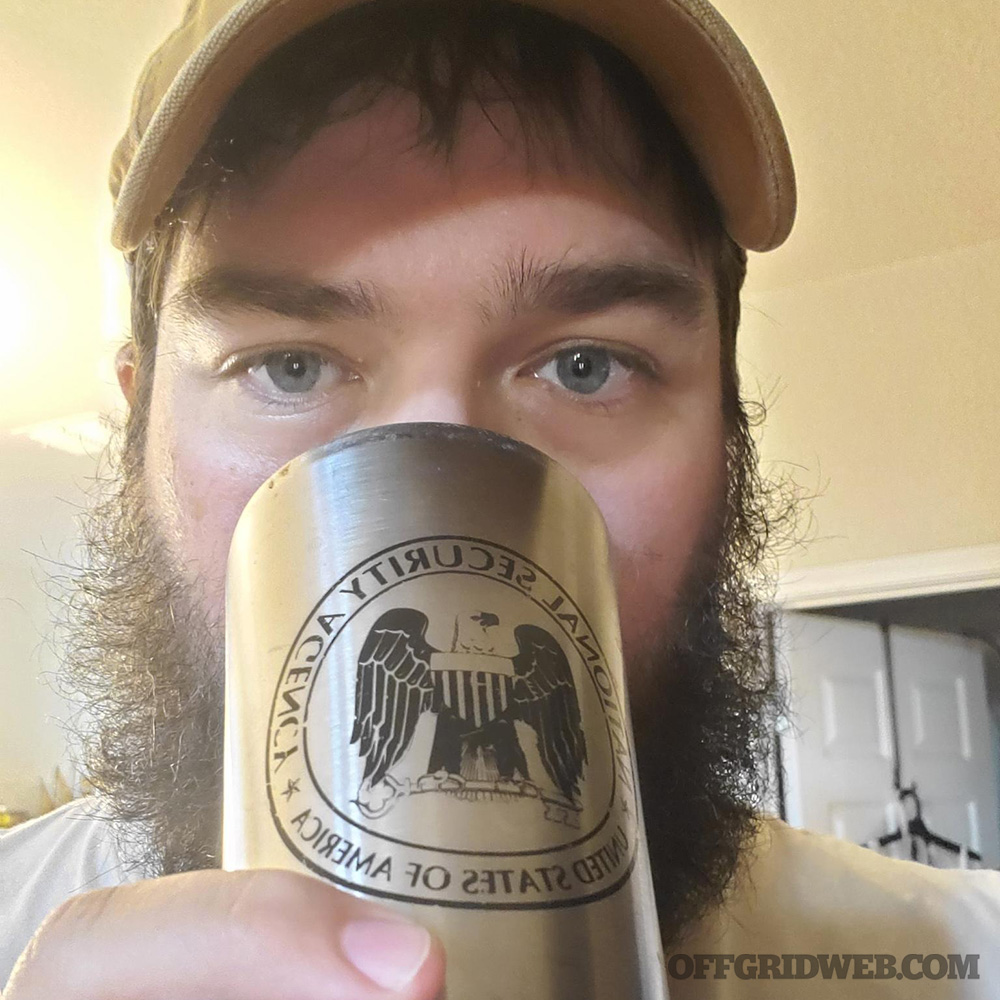
Above: Despite obscuring a large portion of my face with this mug, A match was still made at a distance of an average distance of 0.53, which is near the threshold but still a match. A more sophisticated model would likely defeat this.
Real-World Countermeasures
Broadly speaking, there are three different categories of countermeasure we can use against facial recognition systems in the wild:
- The first type of countermeasure attacks the ability of a system to detect a face in the first place. This is going to include anything from simple face coverings to purpose-driven clothing.
- The second type of countermeasure is going to cause a false negative with facial recognition, after facial detection has occurred.
- The third type of countermeasure is going to attempt to cause a false positive, making the system think that we’re someone else entirely. We’ll call this the “Mission Impossible” countermeasure.
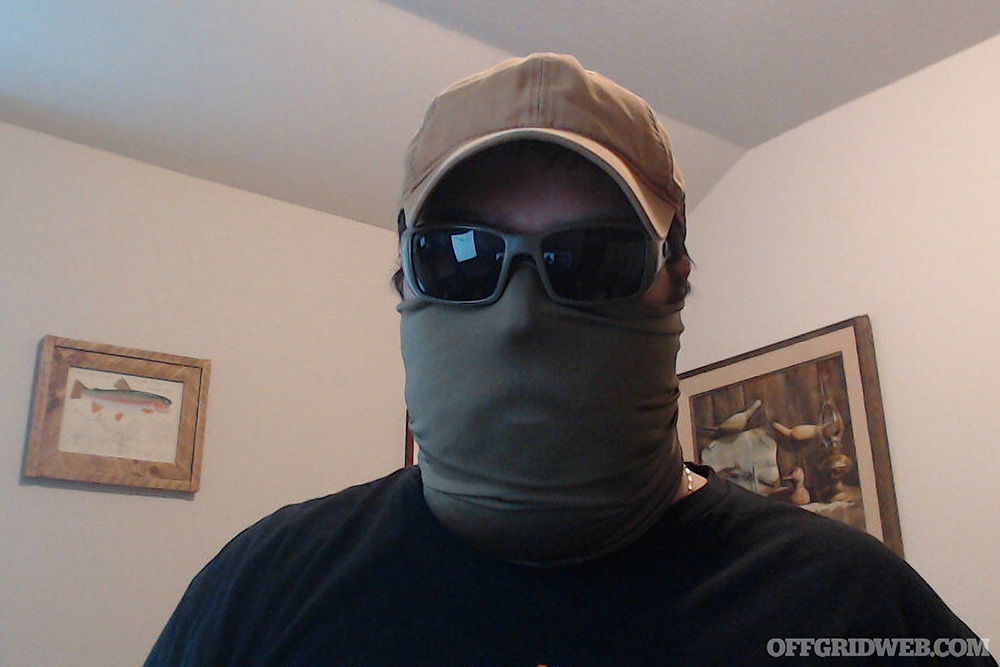
Above: This disguise is fully defeated facial detection, however the utility of wearing something like this in your daily life is kind of a crapshoot.
Countermeasures you can use in the real world are a tricky topic, as it’s very difficult to know with any certainty what will or will not work against any given model. In a general sense, we can be assured that if simple facial detection and recognition models, such as the open-source Python library and tool “facial_recognition” cannot be tricked, then more comprehensive systems powered with sophisticated AI models will also be immune to countermeasures.
To test various types of countermeasures against a baseline, I used the Python tool facial_recognition, which can be installed on any Linux, Mac, or Windows computer with Python on it. This tool will compute the probability of a match in terms of distance from a known baseline, which is to say a smaller number equals a closer match. By default, anything that is 0.6 or higher is considered to not be a match.
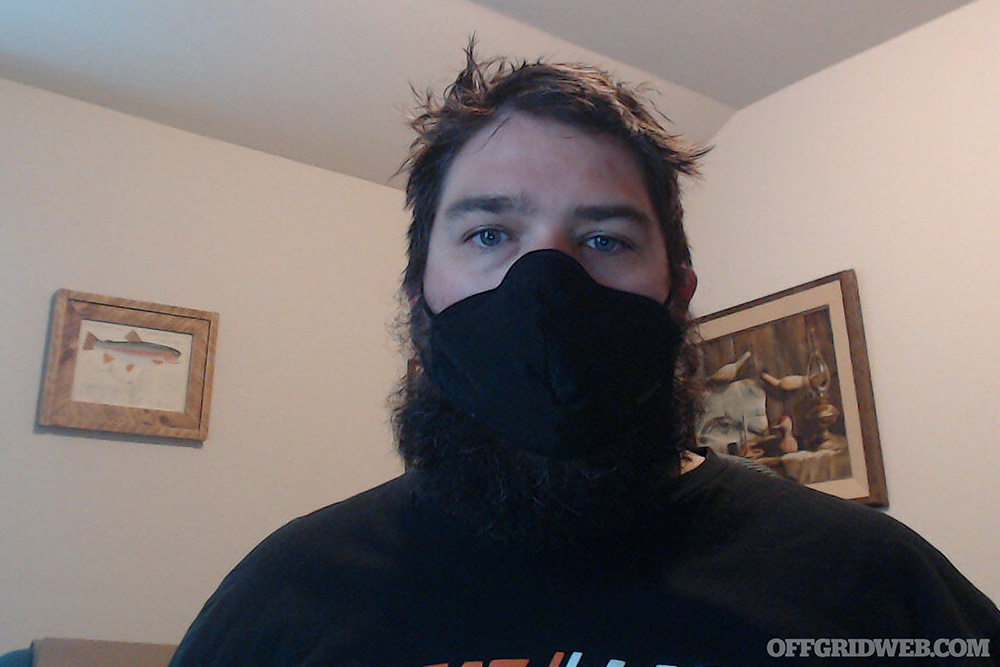
Above: This mask prevented a facial detection, even preventing me from using it as a “known” photo. However, it wouldn’t be enough to counter the surveillance technology used in places like China and shouldn’t be relied on.
For control data, I used the same photo of myself as a known test photo, as well as test photos containing Ukrainian President Zelenskyy and Burt from the move Tremors. A distance of 0.0 was computed when the tool saw the same picture side-by-side. It also correctly determined that I am not Zelenskyy or Burt.
Countermeasure Test Results
So, how did the countermeasures fare?
By far, the best countermeasures were ones that targeted facial detection. Full-face covering of an FDE neck gaiter, wrap-around sunglasses and hat prevented any match. A simple black cloth COVID mask was also enough to prevent any face from being detected, however, this should not be considered reliable as Apple’s iPhone is known to be able to make a conclusive match on data points not covered by a mask, when the user is wearing a mask, so long as a masked photo has been enrolled. This tool, however, couldn’t detect a face even when providing a masked sample image.
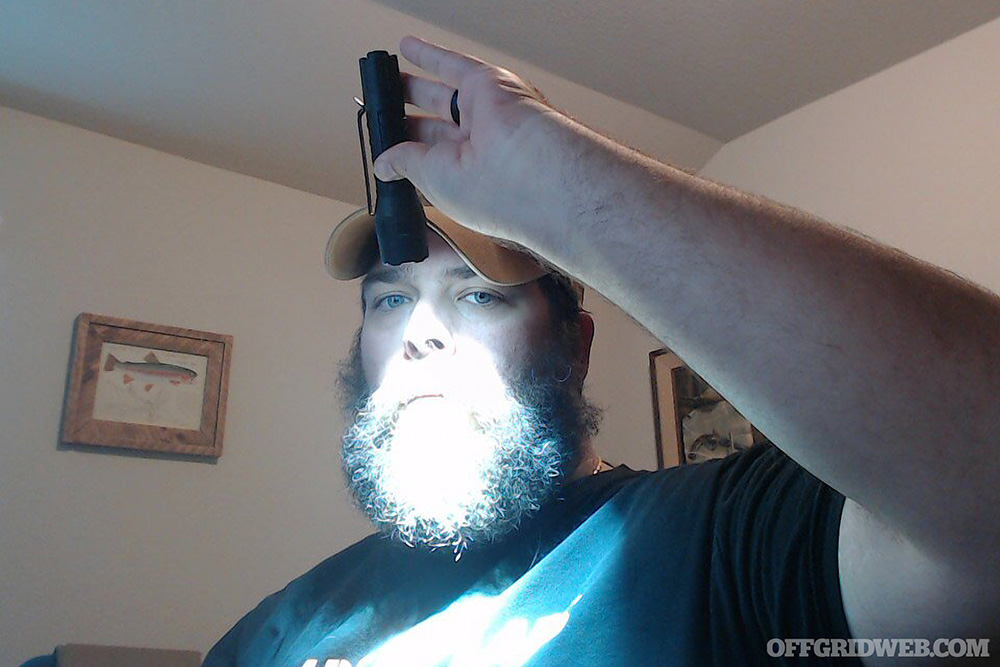
Above: Simulating a hat with a light on it to obscure my face, this was enough to defeat simple open-source facial detection models. The fact I can still recognize myself leads me to believe that a more sophisticated model might still make a match. I still believe that clothing with visible or IR lights that obscure the face are likely to be effective in many cases.
Illuminating my face with a bright flashlight in such a way that it washed out my features also prevented a face from being detected, lending credence to so-called “Liberty Caps” and other such clothing which contain LEDs to obscure the face from cameras. It may be advisable to use infrared LEDs, since their light will be invisible to the human eye while remaining effective against many camera sensors.
“Disguises” meant to obscure my identity, but not completely obscure the face, had mixed results. Predictably, matches became better as more “known” images were added. Thus, merely wearing a hat and glasses would cause a low-confidence match against an “driver’s license” type photo of me, but once photos of myself with hats, glasses, etc., in various combinations were added, the confidence of the matches became closer. However, there was nothing more conclusive than about 0.25. The use of camouflage face paint in the typical application used to obscure lines and flatten the face (darker colors on higher points, lighter on lower points) reduced the confidence of the match but wasn’t sufficient to completely avoid a match.
Digitally obscuring photos with ObscuraCam did prevent matches from being made as well, which proves that such digital countermeasures are effective against non-real-time facial recognition dragnets such as may be conducted using OSINT sources.
“Mission Impossible” disguises weren’t tested. I have low confidence in sophisticated systems being tricked by anything like a Halloween mask. High-end disguises built out by professional makeup artists may be able to make this work, but I think a false negative is going to be the best-case outcome in the general case at this point in the evolution of the technology.
Conclusion
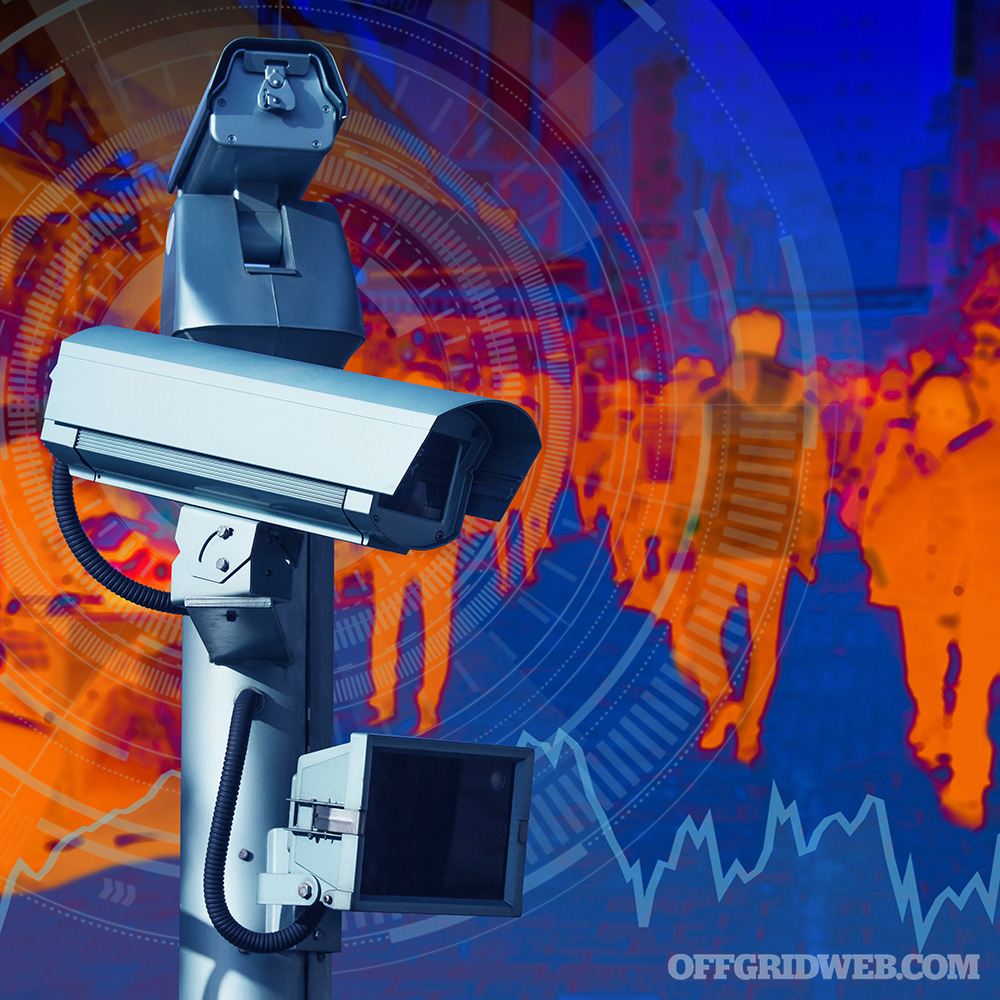
Facial recognition technology is here to stay, and with profound implications for society in general. Just like any other biometric system, there are certainly uses that bring a legitimate advantage, but there’s also an exceptional opportunity for abuse.
Privacy-minded individuals have options to reduce our exposure, but it’s an arms race like any other. While we in the West enjoy legal protections that those in places like China do not, we have far less protection from the monetary drivers of surveillance capitalism that pervade online spaces and the government’s outsourcing of functionality to the private sector to avoid 4th Amendment challenges. In the long run, it’ll become increasingly more difficult to avoid these systems.
Judicious curating of your likeness online can go a long way to limiting the scope of the threat, and there are simple technical countermeasures that can be applied in the real world as well. These steps may not be perfect, but they’re the best we have right now.
Hopefully, this is one topic where civil libertarians will not let up in fighting the potential for abuse. However, as we continue to see facial detection and recognition technologies integrated into people’s daily lives, the expectation of privacy and fear of abuse will winnow over time. Sadly, facial recognition and biometric surveillance will become another “if you’ve done nothing wrong then what are you hiding?” argument in the next generation.
Thus, the best chance we have is in raising a next generation that’ll be resistant to mass surveillance and tracking, and who will adopt appropriate OPSEC as well.
Related Posts
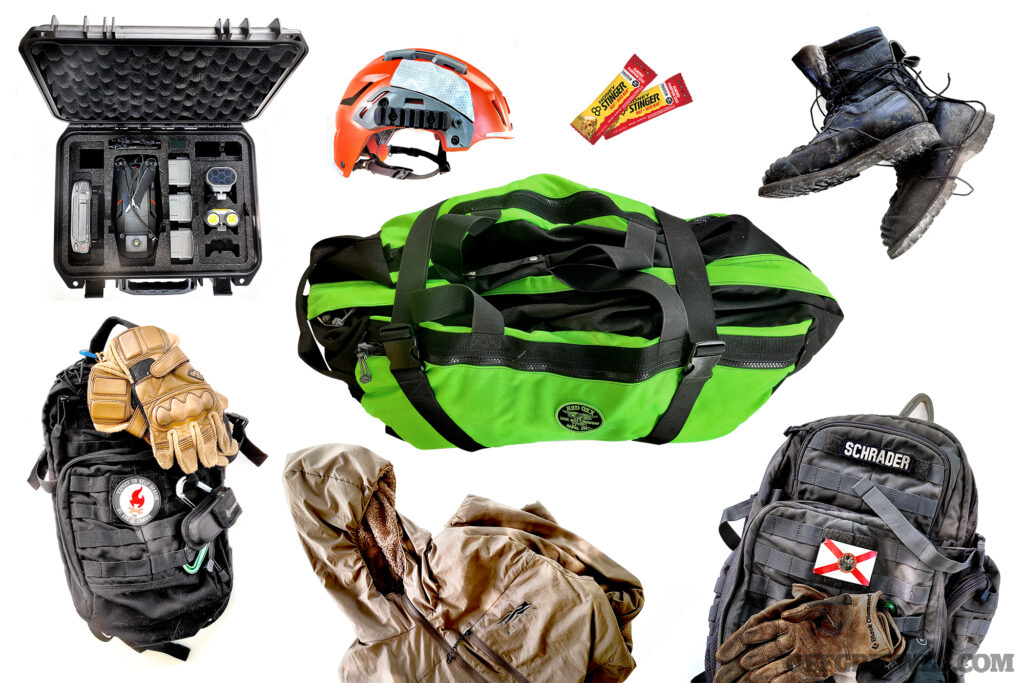
Bag Drop: Red Oxx Search and Rescue Duffel BagThe Red Oxx Manufacturing Sherpa Jr. serves as a “mothership” to my smaller search and rescue bags and keeps my bulky gear all in one place.
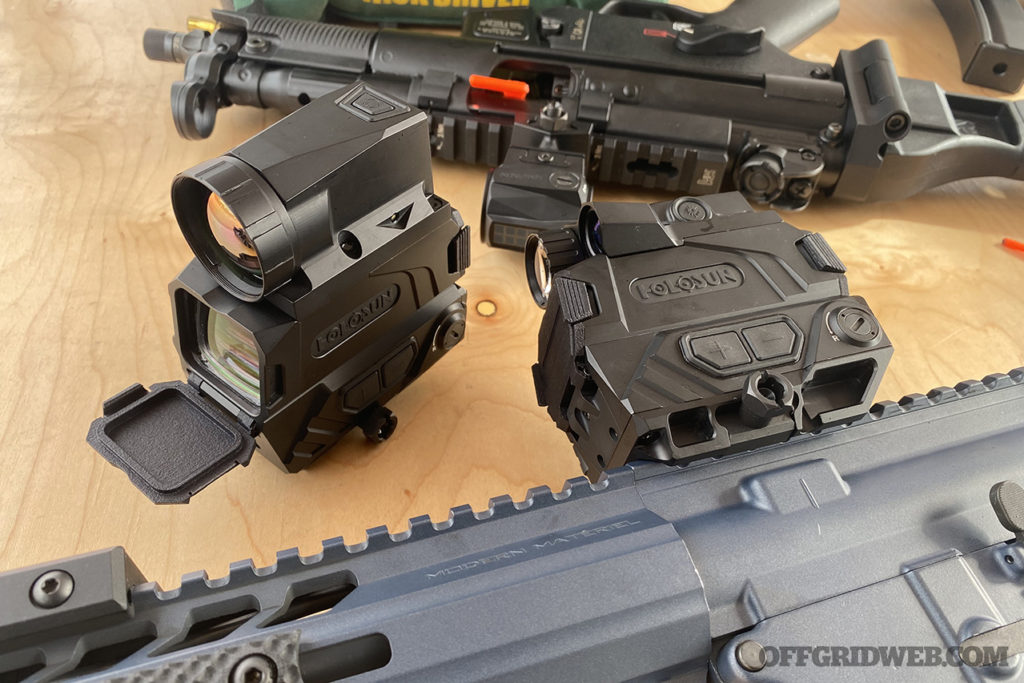
Holosun DRS: New Red Dot + Thermal Hybrid OpticThe new Holosun DRS-TH and DRS-NV optics feature an AEMS-style red dot with a secondary thermal or digital night vision overlay function.
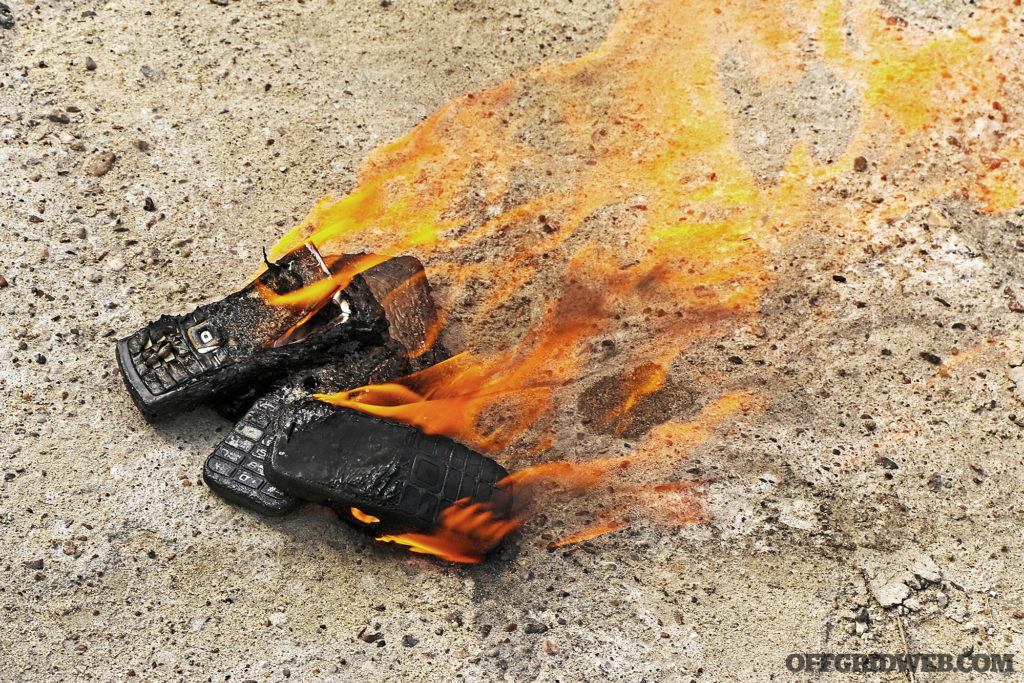
Burner Phone Basics: How to Set Up an Anonymous Prepaid PhoneToday, threats to privacy abound. Those of us who require anonymity can still get it through the use of a prepaid, disposable burner phone.
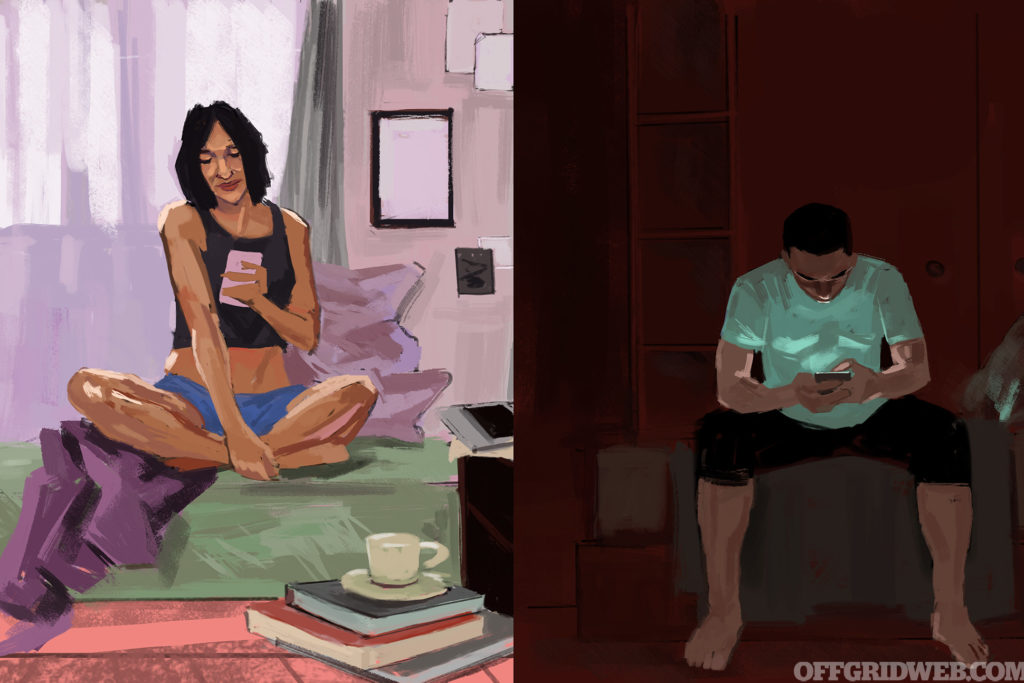
What If You Met Someone Dangerous on an Online Dating Site?In the world of online dating, you have to make safety your number-one priority. People with honest intentions will respect that.
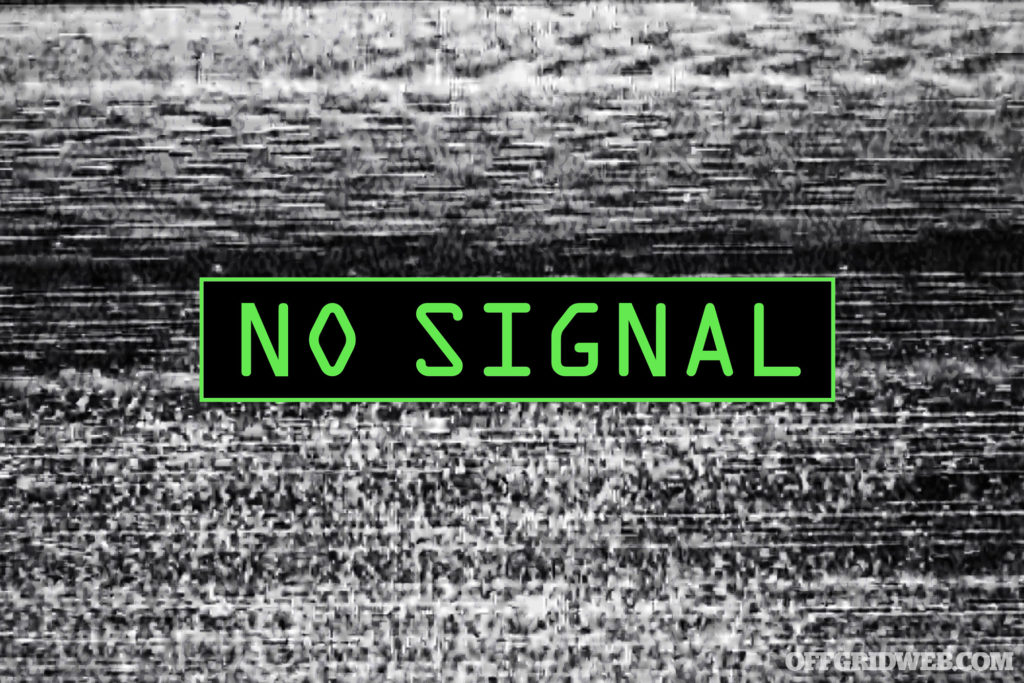
Letter from the Editor: Glitches in the MatrixToday, we live under an ever-increasing burden of technology. It seems as if, everywhere we turn, it plays a greater role in our daily lives.
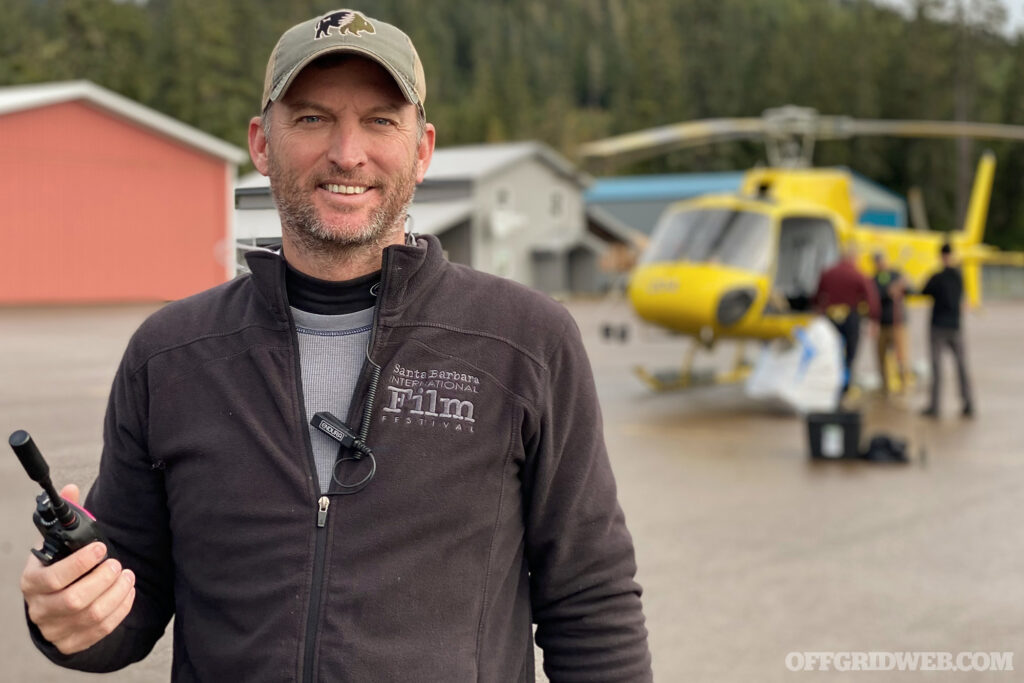
The Making of Outlast: Our Q&A with the Executive ProducerWe spoke with series Executive Producer Grant Kahler regarding the filming of Netflix Outlast and what it takes to survive the wilderness.
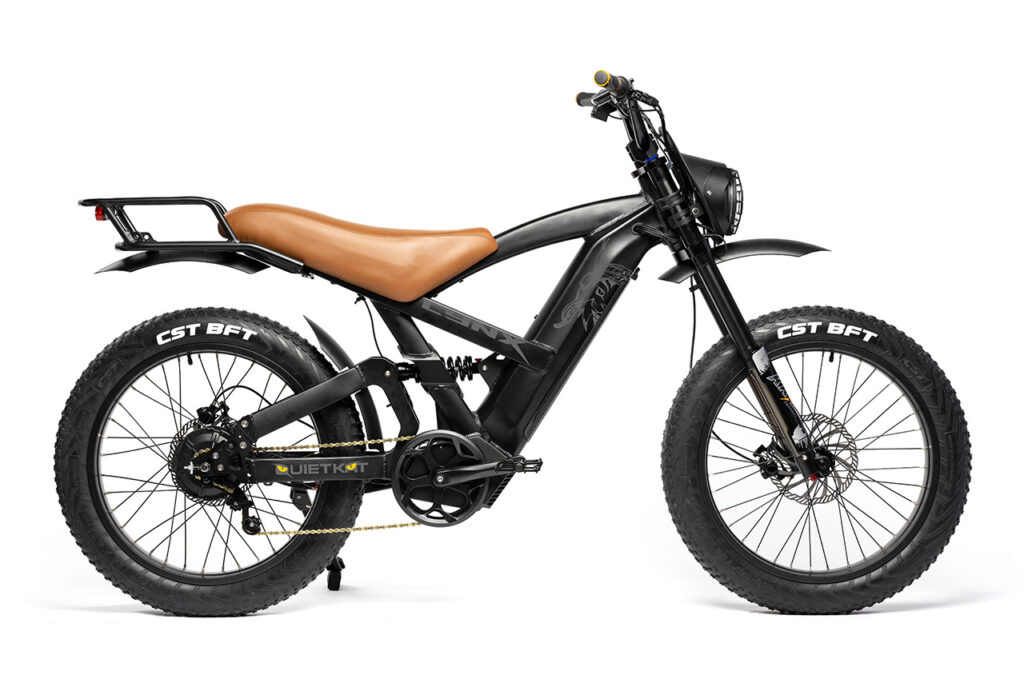
New: QuietKat Lynx eBikeUnveiled last month, the QuietKat Lynx is said to "push the envelope of performance" with a 2-speed, 1000-watt hub motor and twist throttle.
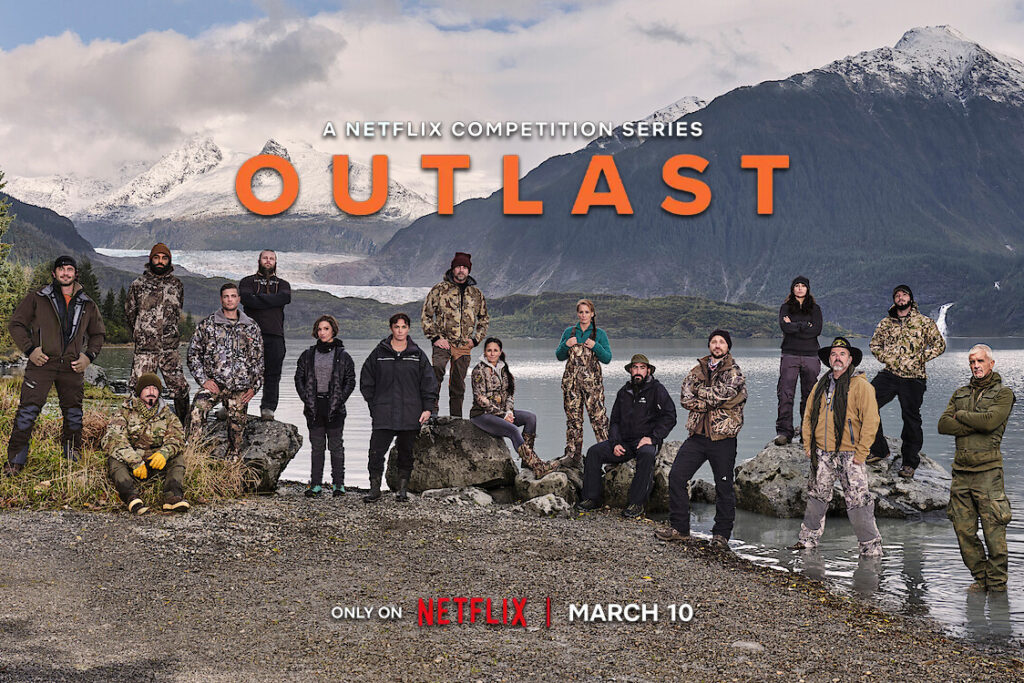
Outlast on Netflix: New TV Show Blends “Alone” with “Lord of the Flies”A new Netflix series called Outlast splits 16 contestants into teams to see who can survive longest and claim a million-dollar cash prize.
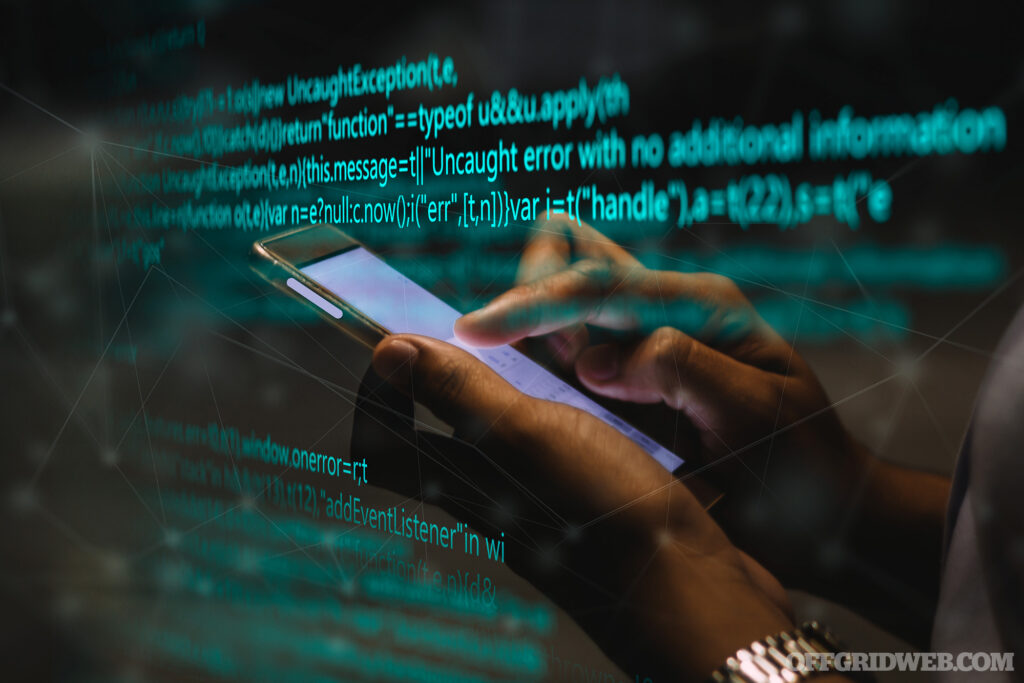
Secure Messaging Apps: Signal, Keybase, Threema, & MoreThere are many secure messaging apps out there, but many are beholden to big-tech oligarchs who don’t value your individual freedoms.
The post Stop Facial Recognition: Countermeasures for Mass Surveillance appeared first on RECOIL OFFGRID.
By: Offgrid Staff
Title: Stop Facial Recognition: Countermeasures for Mass Surveillance
Sourced From: www.offgridweb.com/preparation/stop-facial-recognition-countermeasures-for-mass-surveillance/
Published Date: Sat, 25 Mar 2023 00:41:09 +0000
-------------------------------------------------------------------------
Did you miss our previous article...
https://outdoorsnewswire.com/survivalist/financial-planning-for-preppers